14.4.2025
Artificial Intelligence (AI) is no longer just a futuristic buzzword; it’s redefining how businesses operate through AI in decision-making. From analyzing vast amounts of data to providing actionable insights, AI is becoming an indispensable tool for business leaders and team managers.
The question remains: how much should you trust AI in decision-making, and to what extent should you stay directly involved?
Why Decision-Making Needs a Boost?
Decision-making lies at the heart of every business function. Over the years, trends like big data and data-driven management have pushed companies to generate increasingly large volumes of data. While this approach is intended to simplify decision-making by providing valuable insights, it can also complicate the process.
The sheer volume of data is far beyond what the human brain can effectively process for every decision. This is where AI becomes a powerful tool, helping to manage and extract meaningful insights from the data.
Many organizations face challenges like complexity, cognitive bias, and data overload when striving to make informed decisions. AI, with its capacity to process vast amounts of data and minimize bias, provides a powerful solution. It enhances decision-making by making the process faster, smarter, and more efficient.
What can you expect from this guide? We’ll explore:
- How AI helps address common decision-making challenges
- Key areas where AI is transforming decision-making
- Real-world examples of AI in decision making
- Best practices for integrating AI into your workflows
By the end, you’ll feel equipped to bring AI into your organization’s decision-making processes strategically.
Why Use AI in Decision-Making?
In the sections below, we’ll explore the core benefits of using AI in decision-making, the challenges companies may face, and the importance of maintaining human oversight. You’ll also learn how to distinguish between AI-assisted and AI-driven approaches — and why the collaboration between people and machines is key to better outcomes.
What Are the Benefits of AI in Decision-Making?
Let’s take a closer look at the ways AI is reshaping the decision-making process — from improving efficiency to enabling smarter, data-driven insights.
- Improved Efficiency: AI streamlines complex processes like risk assessment and forecasting, allowing organizations to save time and resources. By delivering data-driven efficiency, reduced risks, and automated insights, AI significantly enhances decision-making. These benefits are especially valuable in dynamic industries such as autonomous vehicles, smart manufacturing and logistics.
- Enhanced Accuracy: Data-driven AI minimizes human error while providing valuable insights through advanced algorithms and real-time data. It enables adaptive reasoning, context-aware decision-making, and the ability to tackle cognitive tasks across multiple domains, overcoming the limitations of task-specific systems.
- Scalability: AI excels at processing vast datasets and analyzing intricate scenarios beyond human capability. Advanced AI systems, and the anticipated future Artificial General Intelligence (AGI), promise to handle immense volumes of data from diverse sources with unparalleled efficiency. Although AGI remains a theoretical concept, current AI technologies already exhibit impressive data-processing power within specialized domains, paving the way toward more general, human-like intelligence. This advancement enables faster, more thorough, and data-driven decision-making, unlocking insights on a scale unattainable by humans alone.
What Are Common Challenges in AI in Decision-Making?
Managing decisions at the companies can be overwhelming. Key challenges include:
- Complexity: Decisions often involve multiple variables, scenarios, or stakeholders. With an explosion of data, organizations often struggle to sort relevant insights from noise.
- Siloed decision-making: Different departments may have their own decision-making processes, leading to inconsistent or conflicting decisions.
- Human Bias: Human cognitive biases may influence choices, whether it’s favoring familiar options (status quo bias) or overly weighing recent events (recency bias).
- Time pressure: In business, time is money. Quick and accurate decisions are essential for maintaining a competitive edge.
- Limited data visibility: Due to data silos or outdated systems, decision-makers may not have access to all the relevant information needed for making informed choices.
What Are Key Challenges in AI-Supported Decision-Making?
While AI offers powerful advantages, it also introduces new challenges that organizations must navigate carefully to ensure ethical yet efficient decision-making.
- Lack of Transparency: Many AI systems are “black boxes,” making their decisions hard to interpret and explain.
- Bias in Data and Algorithms: AI can reinforce existing biases if trained on unrepresentative or flawed data.
- Over-Reliance on AI: People may trust AI decisions too much, even when they lack clarity or context.
- Limited Contextual Understanding: AI struggles with complex human factors like ethics, emotions, and cultural nuances, which is why collaborative decision-making with human involvement remains essential in many situations.
- Accountability Issues: It might be unclear who is responsible for AI-driven decisions — especially when outcomes go wrong.
AI-Assisted vs. AI-Driven Decisions
It’s critical to differentiate between AI-assisted and AI-driven decisions:
- AI-assisted decisions give humans recommendations or insights based on data analysis. Think tools like dashboards or suggestion engines. Humans make the final call of the decisions.
An example: A factory maintenance team uses AI to predict when a machine is likely to fail based on sensor data. The team reviews the prediction and decides when and how to schedule maintenance, considering production plans and resources. So, humans make the final decision.
- AI-driven decisions, on the other hand, allow AI systems to act autonomously within defined parameters.
An example: An automated production line uses AI to adjust temperature or speed settings in real time based on material flow and sensor feedback . All this happens without human involvement, often faster than the humans could.
For critical business functions, AI can enhance decision-making, but human judgment ensures that those decisions are ethical, responsible, and aligned with broader societal values. This human-AI collaboration helps reduce risks and improves outcomes.
What Are Key Areas Where AI in Decision-Making Provides the Most Value?
AI’s impact is most visible where data is abundant, decisions are time-sensitive, and optimization can drive clear results. Below are some key areas where AI in decision-making tends to offer significant value across various industries.
1. Evaluating Options and Optimizing Operations
- Manufacturing and Industry: AI helps in selecting optimal production parameters, improving quality control, and scheduling predictive maintenance.
Example: Choosing when and how to service machines to reduce downtime. - Customer Service: AI supports decision-making in interaction handling, routing queries, and personalizing support.
Example: Deciding whether to escalate a customer issue to a human agent.
2. Risk Management and Real-Time Response
- Energy Management: AI enables real-time monitoring of energy usage, preventing overloads and failures.
Example: Adjusting energy distribution during high-demand periods. - Logistics and Supply Chain: AI manages risks like delivery delays, inventory shortages, or supplier issues.
Example: Automatically rerouting shipments based on real-time traffic or weather data.
3. Forecasting and Scenario Planning
- Education: AI predicts learning outcomes, supports curriculum adjustments, and simulates progress paths.
Example: Identifying students at risk of underperformance early. - Logistics and Supply Chain: AI forecasts demand fluctuations, lead times, and inventory turnover.
Example: Planning stock levels ahead of seasonal peaks. - Energy Management: AI forecasts energy consumption and simulates load scenarios for infrastructure planning.
Example: Anticipating future electricity demand in urban areas.
Best Practices for Integrating AI into the Decision-Making Process
As AI becomes more accessible, companies are exploring how to bring it into everyday decision-making. But effective integration requires more than just plugging in a tool — it’s about aligning AI with real business needs and human workflows. Here are best practices inspired by current research and real-world use cases:
1. Start with AI-Assisted, Not AI-Driven Decisions
Don’t delegate full control to AI. Instead, use it to support human judgment: let AI surface patterns, options, or risks — but keep the final say human. This is ideal for strategy sessions, planning meetings, or project reviews.
2. Prioritize Transparency with Explainable AI
Trust is critical. Choose AI tools that show how they reached their suggestions. Look for features like confidence scores, rationale breakdowns, or alternative scenario comparisons. Explainability builds confidence and makes the decision process more accountable.
3. Use AI Where Humans Struggle With Scale or Complexity
AI shines in data-heavy environments. Good starting points include forecasting (e.g. sales, demand, or traffic), risk modeling (e.g. financial, supply chain, or customer churn), and optimization (e.g. scheduling, inventory, or workflows).
4. Test in Simulated or Low-Risk Environments First
Before rolling out AI across your organization, use “sandbox” projects or limited-scope pilots to evaluate impact and usability. This helps teams build confidence and spot process gaps early.
5. Keep Humans in the Loop for Ethical and Strategic Oversight
Some decisions require human nuance and responsibility. Avoid full automation in ethically sensitive or customer-facing decisions. Use AI to augment, not replace, critical thinking — especially where stakes are high.
6. Support Real-Time Analysis and Action
In fast-paced environments like logistics or operations, use AI to process real-time inputs and recommend or trigger timely actions. It’s especially effective when rapid decisions are needed and data changes constantly.
By integrating AI thoughtfully, businesses can make faster, smarter, and more confident decisions — without losing the human insight that matters most.
Potential Pitfalls to Avoid When Using AI in Decision-Making
While the benefits are substantial, businesses must be cautious of these challenges:
Lack of Explainability (“Black Box” Problem)
Many AI models, especially deep learning systems, are notoriously difficult to interpret. This lack of transparency can undermine trust among users and stakeholders, particularly when the rationale behind a decision cannot be clearly explained. In sectors like finance, healthcare, and law — where decisions carry significant ethical or legal weight — this opacity makes it difficult to justify outcomes or conduct proper audits.
Bias in Data and Algorithms
AI systems often reflect the biases present in their training data. If the data is unrepresentative or skewed, the AI may produce unfair or discriminatory results. Without strong oversight, these biases can be amplified when deployed at scale, negatively impacting areas such as hiring practices, loan approvals, and access to services — often in ways that go unnoticed until real harm is done.
Over-Reliance on AI Outputs
There is a growing risk that users will place too much trust in AI-generated recommendations, accepting them at face value without questioning their accuracy or understanding their limitations. This over-reliance can result in poor decisions, especially in situations where the AI lacks contextual awareness or produces erroneous outputs.
Lack of Moral and Contextual Judgment
Unlike human decision-makers, AI lacks the ability to understand complex emotional, social, and cultural factors. It cannot interpret ethical subtleties or exercise empathy, which makes it unsuitable for decisions that require moral reasoning or human sensitivity. In such cases, relying solely on AI may lead to outcomes that are technically sound but socially tone-deaf or ethically questionable.
Accountability Ambiguity
When something goes wrong in an AI-influenced decision, determining who is responsible can be complicated. Is it the developer who created the algorithm, the business deploying it, or the end user? This lack of clear accountability raises significant legal and ethical concerns, especially when the consequences of a decision affect people’s lives or livelihoods.
Data Quality and Representativeness
The effectiveness of AI depends heavily on the quality of the data it is trained on. If the data is outdated, incomplete, or biased, the resulting decisions may be inaccurate or misleading. Poor data quality can distort predictions and recommendations, reducing the reliability of AI tools and potentially leading organizations down the wrong path.
Misuse or Misapplication of AI Tools
Not every problem is best solved with AI, yet the temptation to apply it broadly persists. Using AI in domains where it lacks sufficient domain knowledge or where high interpretability is required can backfire. There is a real risk of deploying AI simply because it’s available or trendy, rather than because it’s the right fit for the problem — a misstep that can undermine effectiveness and trust.
These pitfalls underscore the importance of human oversight, ethical standards, and clear governance when integrating AI into decision-making processes.
Key takeaways
- AI enhances decision-making by processing large data sets, reducing bias, and improving speed, especially in complex business environments.
- AI-assisted decisions empower humans with insights, while AI-driven systems act autonomously — the right balance depends on the use case.
- Best-fit areas for AI include operations optimization, risk management, and forecasting across sectors like manufacturing, logistics, and energy.
- Integration best practices include starting small, ensuring explainability, keeping humans in the loop, and focusing on high-impact use cases.
- Key risks involve lack of transparency, data bias, over-reliance, ethical blind spots, and unclear accountability — making oversight essential.
Source and further reading:
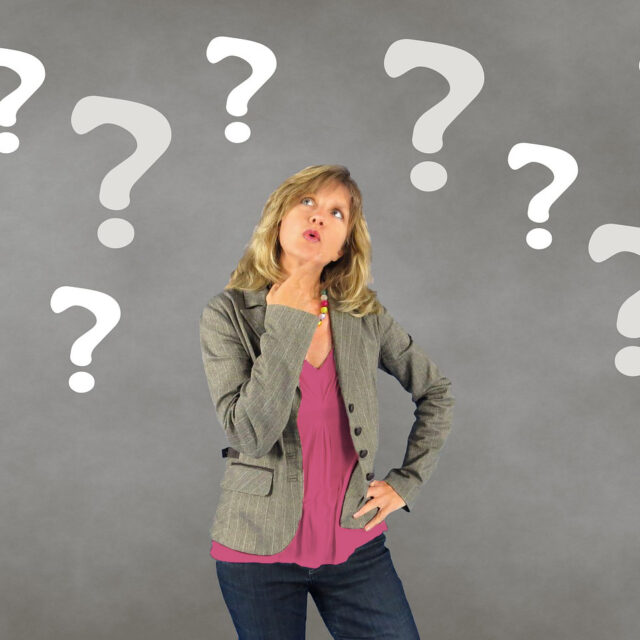
Ready to Make Smarter Decisions Together?
Try Noni — a real-time, collaborative decision-making tool designed to combine team input with clarity and structure.